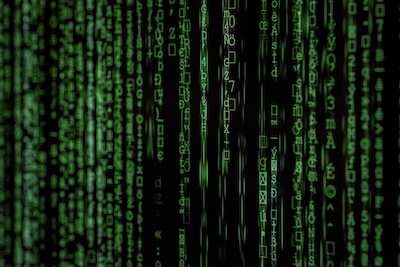
Data Quality: A Part of Corporate Culture
CerebraQuality and efficiency of data analysis process largely depends on “data quality”. Today’s trend of enlightenment on the importance of data and high expectations from data analysts to add value to an organization remains unsupported without accurate, complete, consistent, valid, unique and timely data structure.
Data is now considered as one of the core enterprise assets of an organization. Having the right data at the right time to make the right decision is not a benefit, but a crucial component of organization’s success or failure. Therefore, the information gathered by analyzing the data, should be appreciated, managed and leveraged by all organizations to gain maximum competitive advantage.
As raw material, data is distinctly different from its counterparts in process of manufacturing. Information is not a “use once” commodity; it can be cloned, moved, transformed and repurposed in data analysis whereas data is only useful when it is of high-quality. “Right first time” and “fitness for use” are two popular phrases to describe the art of quality assurance.
Improved data analysis supported by prime data leads to better decision-making across the organization, better audience targeting, more effective content and marketing campaigns, improved relationships with customers, easier implementation of data, competitive advantage and last but definitely not least, increased profitability.
The impact of data quality on the information chain has been widely recognized since the introduction of large-scale data processing and data analysis. Furthermore, the nature and usage of data themselves remarkably changed because of big data, high accessibility leading to unprecedented distribution and sharing of data, and lack of match between the intention of data creation and its subsequent usage in data analysis in recent years.
A proactive approach to data management aligns data quality along a number of quality dimensions:
Accuracy: It is the most visible and significant dimension of data quality and it is defined as recorded data’s conformity to actual value. A complete and 100% accuracy may not be attainable, but through a process of continuous improvement, defects are eliminated, new features are identified, and any pervasive behavior is monitored during the data’s lifecycle.
Completeness: It is defined as expected comprehensiveness. As long as the data meets the expectations then the data is considered complete.
Consistency: It means data across all the systems reflects the same information and is synchronized across the enterprise. It is the absence of difference, when comparing two or more representations of a thing with a definition.
Validity: Data is valid if it conforms to the syntax (format, type, range) of its definition.
Uniqueness: It means nothing will be recorded more than once based upon how that thing is identified.
Timeliness: It refers to the degree to which data represent reality at the required point in time.
Even when all these six dimensions are deemed to be satisfactory, the data can still fail to achieve the required objective. We still need to check the comprehensibility, simplicity, relevancy, accessibility and maintainability at the right level of precision.
Making data quality a priority, automating data entry, preventing duplicates, not just curing them, taking care of both master and metadata are the best practices that can help improve the quality of data.
Companies could not produce a Key Performance Indicator (KPI) around the quality of their data, while some were not even convinced of organizational value of a data quality program. Despite questionable data quality, companies rely on their available data to make their most critical decisions. Organizations began to realize the impact of the “garbage in, garbage out” approach on their costly resources, due to the time spent on non-productive tasks such as searching or trying to fix data errors.
Many organizations initiate projects to clean up “dirty data,” but without a framework for standards, policy and data life cycle management, these projects turn out to be ineffective and fail to resolve the root cause of poor data quality.
Here are some of the reasons of poor data quality:
- Errors are typical in the creation or transportation of data via manual entries, data migration and conversion projects, changes to source systems, mixed entries by multiple users, software updates, inadequate testing time,
- Data quality is not a “sexy topic” compared to concluding a financial transaction or trading,
- People mostly have “I’ll fix it myself in Excel” approach to getting things done,
- Creation of silos and tendency of departmental approaches focusing on data integration, which is often resulted by each department “having its own copy” of the data,
- Disposition of retired or redundant data is omitted despite the exponential growth in data.
Data is there “to help your organization achieve its goals” so it must be recognized as an enterprise asset with a robust data governance plan. Here are the basic necessities before beginning to implement such a plan:
- Ensure the involvement of top-level management,
- Determine the users of the data and the purpose it serves,
- Use a business glossary as a foundation for metadata management,
- To find a solution for each data quality issue raised, start with a root cause analysis,
- Make a complete gap analysis for your current available data,
- Improve the skills of your employees to gather useful information from the data analysis,
- Be aware of the new environment formed by the increased usage and accumulation of data,
- Be aware of the developments in business intelligence tools to use data in analysis,
- Transform your corporate culture towards a data-driven approach by opening up data access, becoming data literate, aligning data with business objectives.
Data governance is necessary in order to handle data effectively and maintain data quality across the organization. Choosing the best model for the organization, selecting the right team members, and ensuring continued compliance with policies and procedures are the basics to establish an effective and successful program that will improve company’s data for years to come. It is now exceedingly important to disseminate the view that data quality is the responsibility of all the participants in an organization, not just IT.